The Role of Internal Audit in Responsible AI and AI Act Compliance
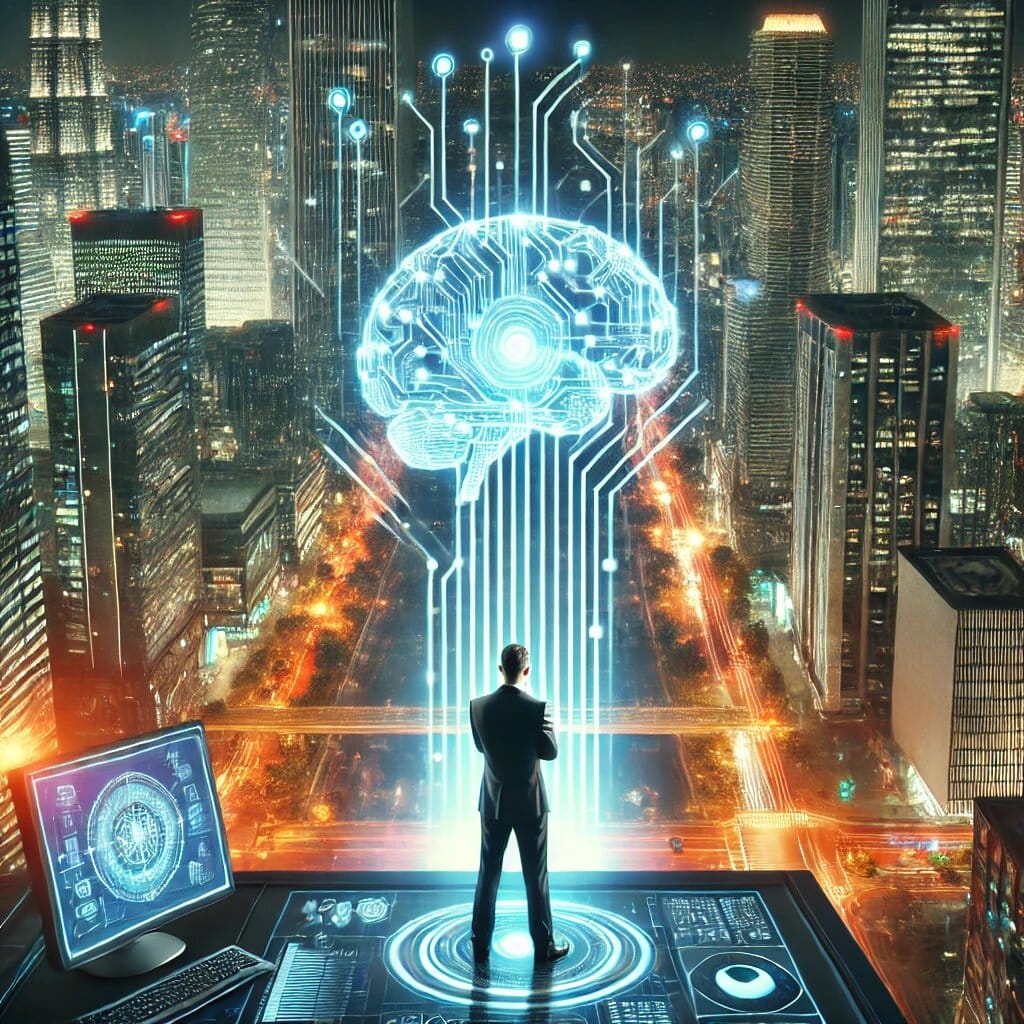
Introduction As Artificial Intelligence (AI) becomes increasingly integrated into organizations, the need for responsible AI practices and compliance with regulations like the AI Act is growing. Internal audit (IA) departments can play a crucial role in guiding organizations toward responsible AI implementation and ensuring compliance.
The AI Safety Report The first International AI Safety Report, created with input from a diverse group of AI experts, emphasizes the importance of managing the risks associated with general-purpose AI while acknowledging its potential benefits. The report focuses on identifying these risks and evaluating technical methods for mitigating them. It also highlights the rapid advancements in AI capabilities and the need for international cooperation in risk management.
The Role of Internal Audit Internal audit departments can assist their organizations with the journey toward responsible AI and compliance with the AI Act. They can develop audit frameworks to assess AI use, provide recommendations to deliver benefits, and reduce risks. IA departments are encouraged to lead by example, even though only a few are currently using AI.
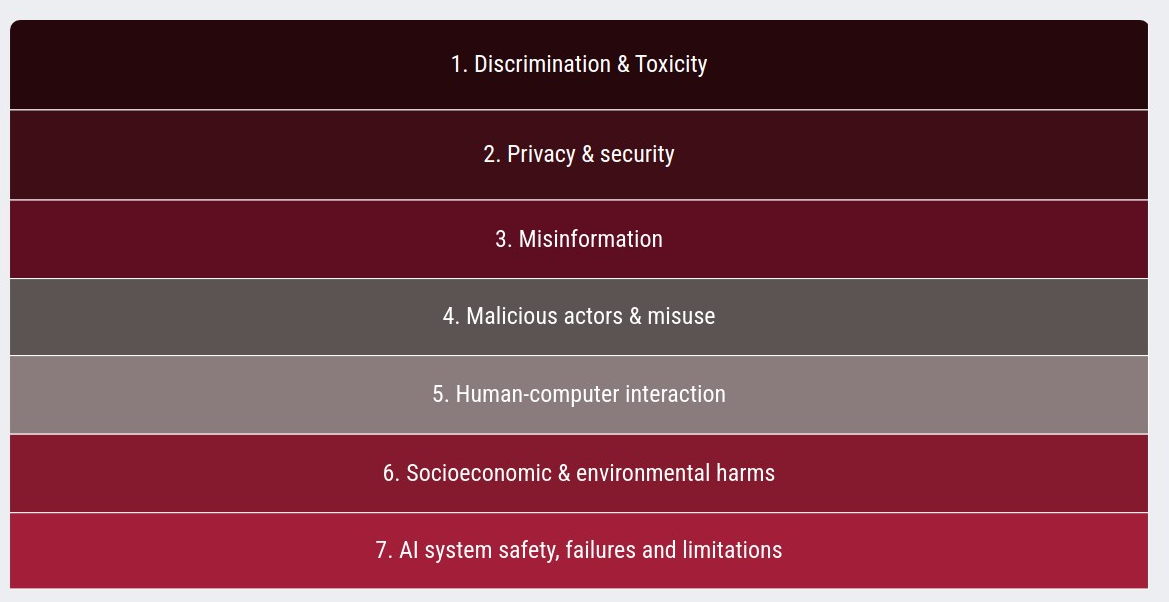
Key areas for Internal Audit Engagement
- Developing Audit Frameworks: IA can create frameworks to evaluate AI applications within their organizations.
- Risk Assessment and Mitigation: Identifying and mitigating risks associated with AI is a critical function of IA.
- Compliance: IA can help ensure their organizations adhere to the AI Act and other relevant regulations.
AI System Lifecycle IA should consider the entire AI system lifecycle, including:
- Development: General-purpose AI models are developed through pre-training, fine-tuning, and system integration. Fine-tuning involves adapting a pre-trained model to a specific task, often requiring feedback from data workers. System integration combines AI models with components like user interfaces and content filters.
- Deployment: This involves implementing AI systems into real-world applications. 'Deployment' differs from 'model release', where trained models are made available for further use.
- Monitoring: Regular monitoring and auditing are essential for identifying and addressing potential issues, ensuring ongoing compliance and effectiveness.
Key Considerations for AI Risk Management
- Data Quality and Governance: High-quality data is essential for effective AI. Data collection methods, storage, and labeling all impact data quality.
- Transparency and Explainability: It is important to provide end-users with insights into how AI systems operate and what their limitations are.
- Human Oversight: Maintaining human involvement in decisions based on AI input is crucial.
- Privacy and Confidentiality: Privacy legislation must be considered throughout the AI system lifecycle. Removing personally identifiable information (PII) from training data is a cost-effective way to reduce risk.
- Reproducibility: Ensuring the AI model produces consistent results and documenting datasets and processes are important aspects.
- Accountability: Defining clear roles and responsibilities for AI systems is essential.
Technical methods for detecting and defending against harmful behaviors
- Anomaly Detection: Anomaly detection can help detect unusual inputs or behaviors from AI systems.
- Defense in Depth: Multiple layers of protection and redundant safeguards can increase confidence in safety.
- Human in the Loop: When there is a high risk of a general-purpose AI system taking unacceptable actions, a human in the loop can be essential.
Addressing Data and Copyright Concerns
- Data Collection: AI developers use web crawlers to navigate the web and copy content, but websites are increasingly blocking these crawlers due to copyright concerns.
- Copyright Infringement: There are legal questions about using copyrighted works as training data. Legal uncertainty has disincentivized transparency about what data developers have collected or used.
- Mitigation Strategies: While technical strategies can mitigate the risks of copyright infringement from model outputs, these risks are difficult to eliminate entirely.
The Importance of Transparency and Documentation
- Transparency: Organizations should be transparent about the design, operations, and limitations of their AI systems.
- Documentation: Standardized documentation can help organizations systematically integrate AI risk management processes and enhance accountability efforts. This includes documenting data generation processes, AI system inventories, and ethical frameworks.
Conclusion Internal audit departments have a significant opportunity to guide their organizations in the responsible and compliant use of AI. By focusing on key areas such as risk assessment, data governance, transparency, and ongoing monitoring, IA can help ensure that AI delivers benefits while minimizing potential risks. Staying informed about evolving AI technologies, risks, and mitigation techniques is essential for internal auditors to provide effective guidance and assurance.