Navigating the AI Regulatory Maze: A Compliance Blueprint for Trustworthy AI
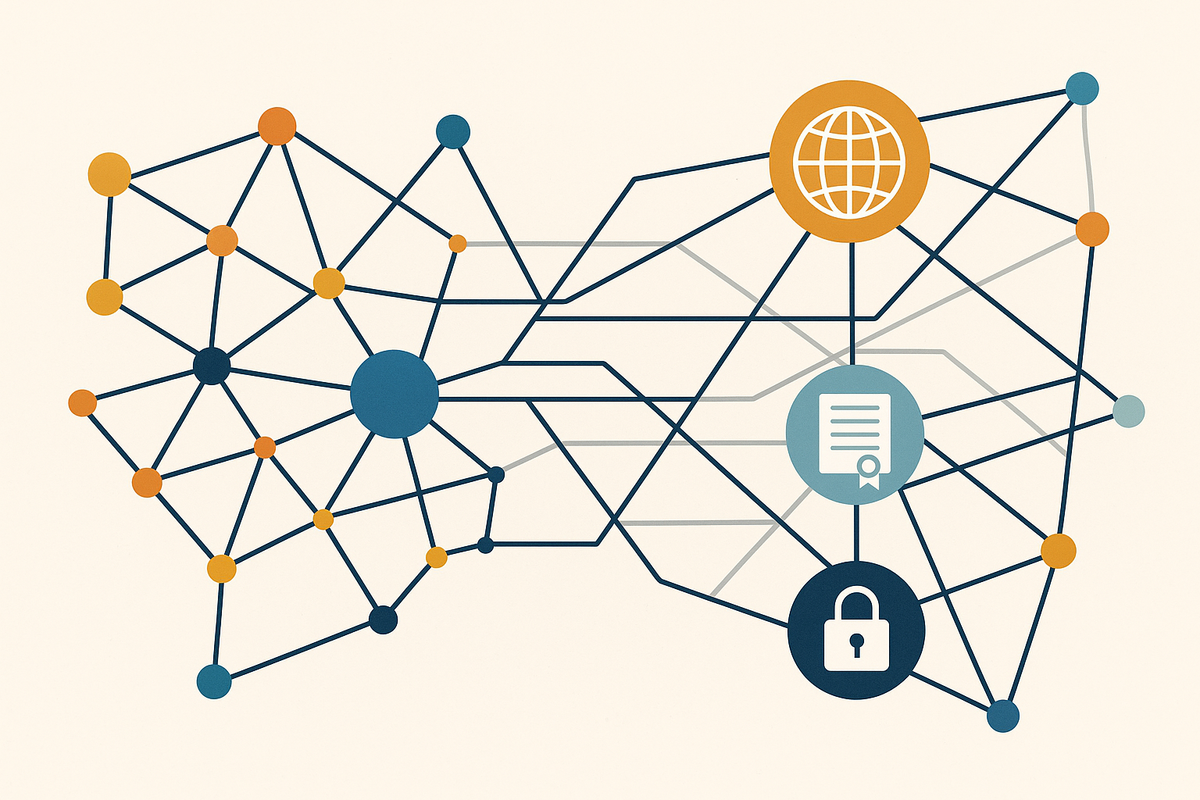
Artificial intelligence is no longer a futuristic concept; it's an integral part of modern business operations. From automating complex tasks to informing strategic decisions, AI promises efficiency and innovation. However, with this transformative power comes a rapidly evolving landscape of legal and ethical challenges. As companies increasingly deploy AI systems, ensuring these systems are not only effective but also trustworthy and compliant is paramount. From a compliance perspective, this isn't just a best practice – it's becoming a fundamental requirement.
The global regulatory environment for AI is developing swiftly, signaling a clear intent from governments to ensure AI is developed and used responsibly. Understanding this dynamic landscape is the crucial first step in building a robust AI compliance program.
The Evolving Global Landscape
The European Union's AI Act stands out as a pioneering, comprehensive legal framework. It takes a risk-based approach, classifying AI systems into different levels: unacceptable risk, high risk, limited risk, and minimal/no risk. This risk categorization directly informs the compliance obligations placed on both providers (developers) and deployers (users) of AI systems.
For AI systems deemed high-risk, the obligations are stringent. These include mandatory requirements such as:
- Implementing and maintaining adequate risk management processes throughout the AI system's lifecycle. This involves continuous risk assessments.
- Ensuring appropriate data governance and management practices for training, validation, and testing datasets to guarantee data quality and prevent discrimination or inaccurate results. Sensitive personal data should generally not be included unless necessary to avoid discriminatory outcomes.
- Creating and maintaining technical documentation that demonstrates compliance with obligations and facilitates conformity assessments.
- Implementing logging of events to ensure traceability of the system's functioning, including minimum logging for usage, data, and personnel identification.
- Ensuring human oversight is possible and appropriate.
- Meeting requirements for accuracy, robustness, and cybersecurity.
- Being registered in the EU database for high-risk AI systems before being placed on the market.
- Complying with transparency obligations to enable correct interpretation and use, providing instructions in an appropriate format.
Systems classified as General-Purpose AI (GPAI) or foundation models also have specific transparency requirements, including technical documentation, compliance with EU copyright law, and providing information on training data. More powerful foundation models face additional obligations like model evaluation, systemic risk assessment and mitigation, adversarial testing, serious incident reporting, and ensuring cybersecurity and energy efficiency. For limited-risk systems, transparency primarily means informing users that they are interacting with an AI system.
The EU AI Act is expected to become law in 2024, with compliance generally expected by 2025, although rules on GPAI become effective in August 2025. Penalties for non-compliance can be significant, potentially reaching millions of euros or a percentage of global turnover depending on the infringement and company size. Its potential extraterritorial effect means organizations outside the EU operating within the EU market must consider its requirements.
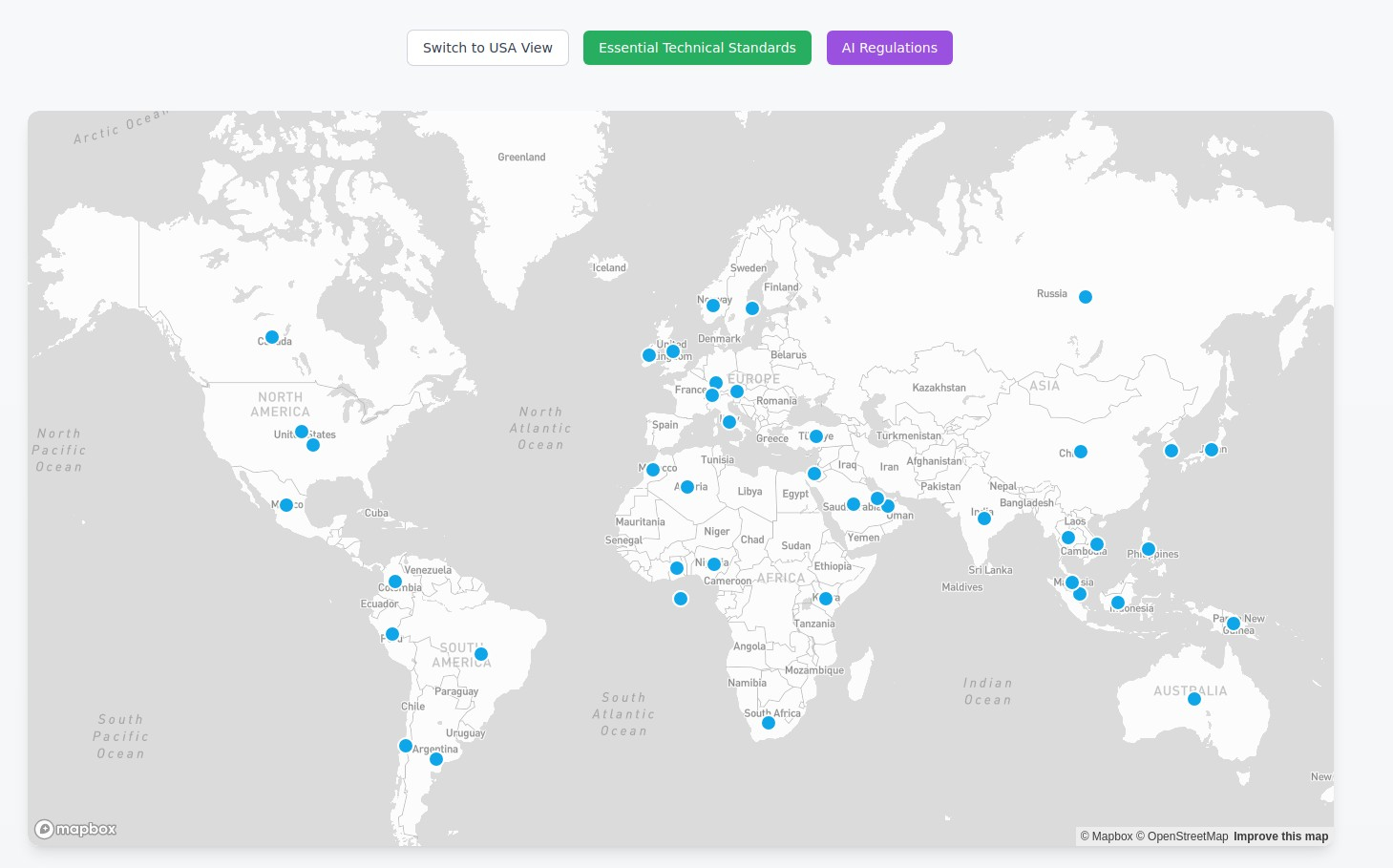
In the United States, the approach is also evolving. While existing laws address some AI risks, new guidance is emerging. A key resource is the voluntary NIST AI Risk Management Framework (AI RMF). The AI RMF is designed to help organizations manage AI risks and promote trustworthy and responsible AI. Although voluntary, it aligns with the risk-based approach seen globally and provides a structured way for organizations to build the practices that will likely underpin future US compliance requirements.
The AI RMF structures AI risk management around four functions: GOVERN, MAP, MEASURE, and MANAGE. From a compliance standpoint:
- GOVERN is foundational, establishing the organizational culture, policies, roles, responsibilities, and accountability needed for a compliance program. It requires integrating AI risk management into broader enterprise risk management. Senior leadership responsibility is key.
- MAP helps organizations identify AI systems and their context, which is essential for determining if a system falls under specific regulatory categories (like high-risk) and identifying the applicable obligations and potential risks.
- MEASURE provides methods to evaluate AI system trustworthiness and identify risks. This is critical for demonstrating compliance through objective, repeatable testing, evaluation, verification, and validation (TEVV) processes.
- MANAGE involves prioritizing risks, implementing controls, and handling incidents. This function directly addresses putting the required compliance controls and processes into practice.

The AI RMF also emphasizes characteristics of trustworthy AI systems, such as being secure and resilient, accountable and transparent, explainable and interpretable, privacy enhanced, and fair with harmful biases managed. These characteristics align closely with the objectives of global AI regulations. The Framework is flexible and intended to augment existing risk practices, aligning with applicable laws and regulations. Profiles can be used to tailor the Framework to specific use cases or sectors, helping organizations align with legal/regulatory requirements and risk management priorities.
Beyond these major frameworks, US efforts include the NTIA's work on AI accountability policy, CISA's focus on AI cybersecurity collaboration and voluntary information sharing, and a growing body of state-level AI governance legislation impacting the private sector. This highlights the need for organizations to track requirements across jurisdictions where they operate or intend to operate.
Building Your AI Compliance Program: Practical Steps
Establishing a robust AI compliance program requires a multidisciplinary effort involving legal, privacy, data science, risk management, procurement, and technical teams. Here are key actions organizations should take:
- Define Governance and Policy: Establish clear policies for identifying AI system risk levels based on categories like those in the EU AI Act. Implement or improve your AI governance framework aligning with regulatory requirements and good practices. Define roles and responsibilities for AI risk management at all levels, ensuring clear accountability.
- Inventory and Classify AI Systems: Conduct a thorough review of existing AI systems and use cases to categorize them by risk level and identify those requiring specific compliance measures.
- Conduct Gap Analysis and Risk Assessments: Compare your current AI practices against applicable regulatory requirements (like the EU AI Act) and frameworks (like the NIST AI RMF) to identify areas of non-compliance. Implement continuous risk management processes to identify, evaluate, and mitigate risks throughout the system lifecycle.
- Implement Controls and Processes:
- Develop and implement data governance practices to ensure the quality, relevance, and legality of data used for training, validation, and deployment. This includes addressing privacy requirements (e.g., GDPR, CCPA) through measures like anonymization or synthetic data where appropriate.
- Prioritize transparency and explainability. Ensure technical teams can provide explanations of how AI systems reach decisions, especially for high-risk systems or those impacting individuals. This is crucial for building trust and demonstrating compliance with anti-discrimination and unfair practice laws. Define internal processes for determining the appropriate level of explainability, considering potential trade-offs with accuracy. Update consumer terms, privacy policies, and consent notices as needed, including 'explainability' statements.
- Establish processes for technical documentation and logging that meet regulatory requirements. Logging should enable monitoring, auditing, and incident response. Consider appropriate logging levels for generative AI systems.
- Ensure robust cybersecurity and resilience for AI systems and their underlying infrastructure. Apply traditional security controls alongside AI-specific mitigations for new threats. Conduct threat modeling using frameworks like MITRE ATLAS and OWASP Top 10 for LLMs.
- Define and implement procedures for human oversight, especially for high-risk applications.
- Manage Third-Party Risk: Enhance third-party risk assessments to cover AI-specific considerations, especially when using foundation models or components from external providers. Verify their compliance intentions and understand the technical documentation they provide to help you manage downstream risks.
- Train Employees: Educate your workforce, including developers, data scientists, and even end-users, on AI ethics, security threats, responsible practices, and specific compliance obligations relevant to their roles. Foster a security and safety-first mindset.
- Establish Monitoring and Incident Response: Implement processes for continuous monitoring of AI system performance, trustworthiness characteristics, and security events. Develop and practice incident response plans specifically for AI-related security incidents and vulnerabilities. Ensure incidents and errors are communicated to relevant stakeholders, including affected communities.
- Engage with Policymakers (Optional but Recommended): Consider participating in industry discussions, policy-making processes, or regulatory sandboxes to stay ahead of trends and provide input.
Compliance is a Continuous Journey
The AI regulatory landscape is not static; it's constantly evolving. A successful AI compliance program is a living system that requires continuous evaluation, adaptation, and improvement. By adopting a proactive and structured approach, leveraging frameworks like the NIST AI RMF to operationalize requirements from regulations like the EU AI Act, and embedding a culture of responsible AI throughout the organization, companies can navigate the complexities of AI governance, build trust, and unlock the full potential of this transformative technology while staying on the right side of the law.
This article is intended for informational purposes and does not constitute legal advice. Organizations should consult with legal and compliance professionals to address their specific circumstances.