Navigating AI Data Compliance: A Technical Overview
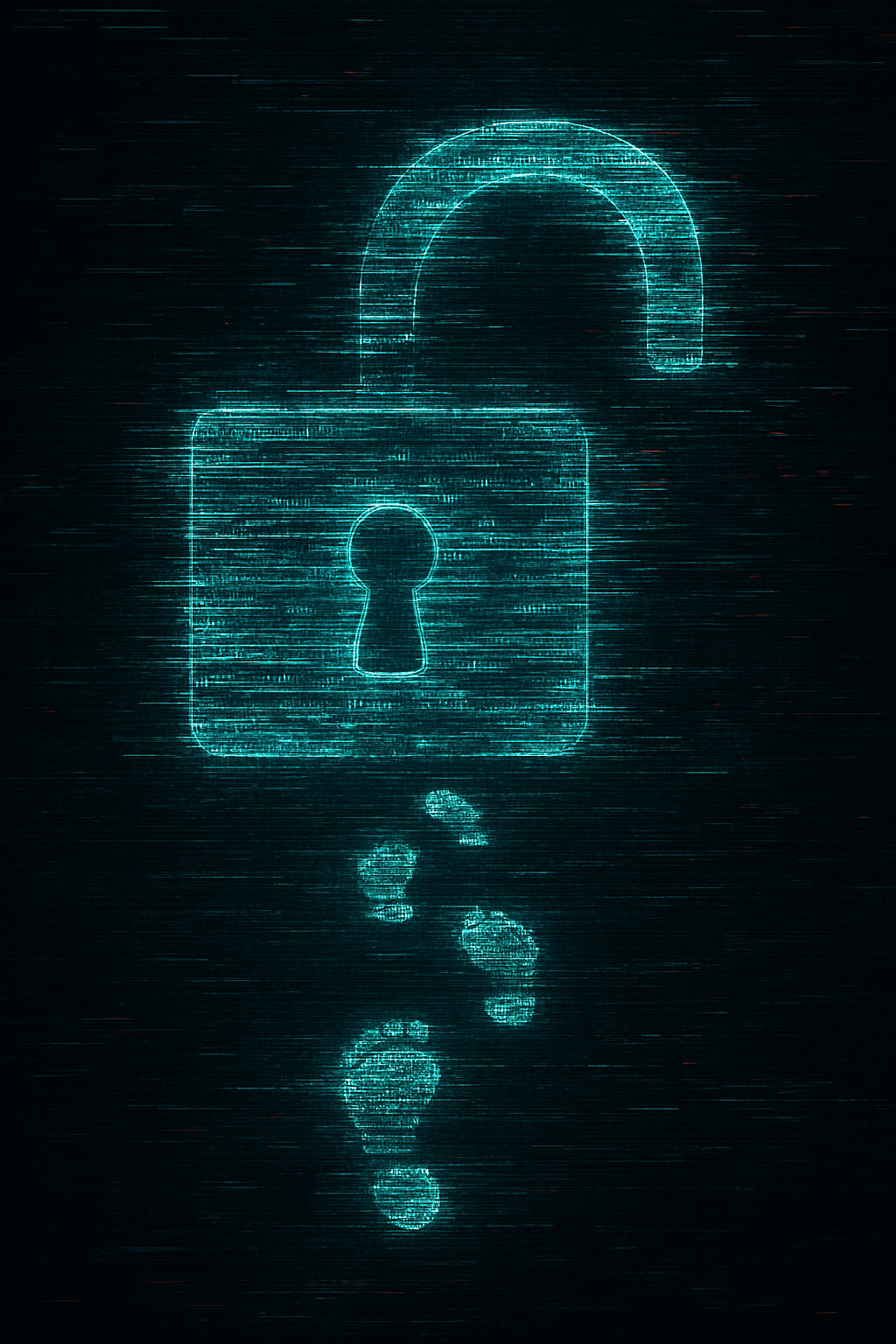
The integration of Artificial Intelligence (AI) into enterprise operations presents transformative opportunities, but it also introduces significant complexities in maintaining data security and achieving regulatory compliance. Organizations must adopt comprehensive security strategies that specifically address the unique challenges associated with AI models, their infrastructure, and the data they process. This article provides a technical overview of key compliance considerations for data within AI ecosystems, drawing from insights in the SANS Critical AI Security Controls and the Google analysis of threat actor interactions with Gemini.
Data Protection: The Foundation of AI Compliance
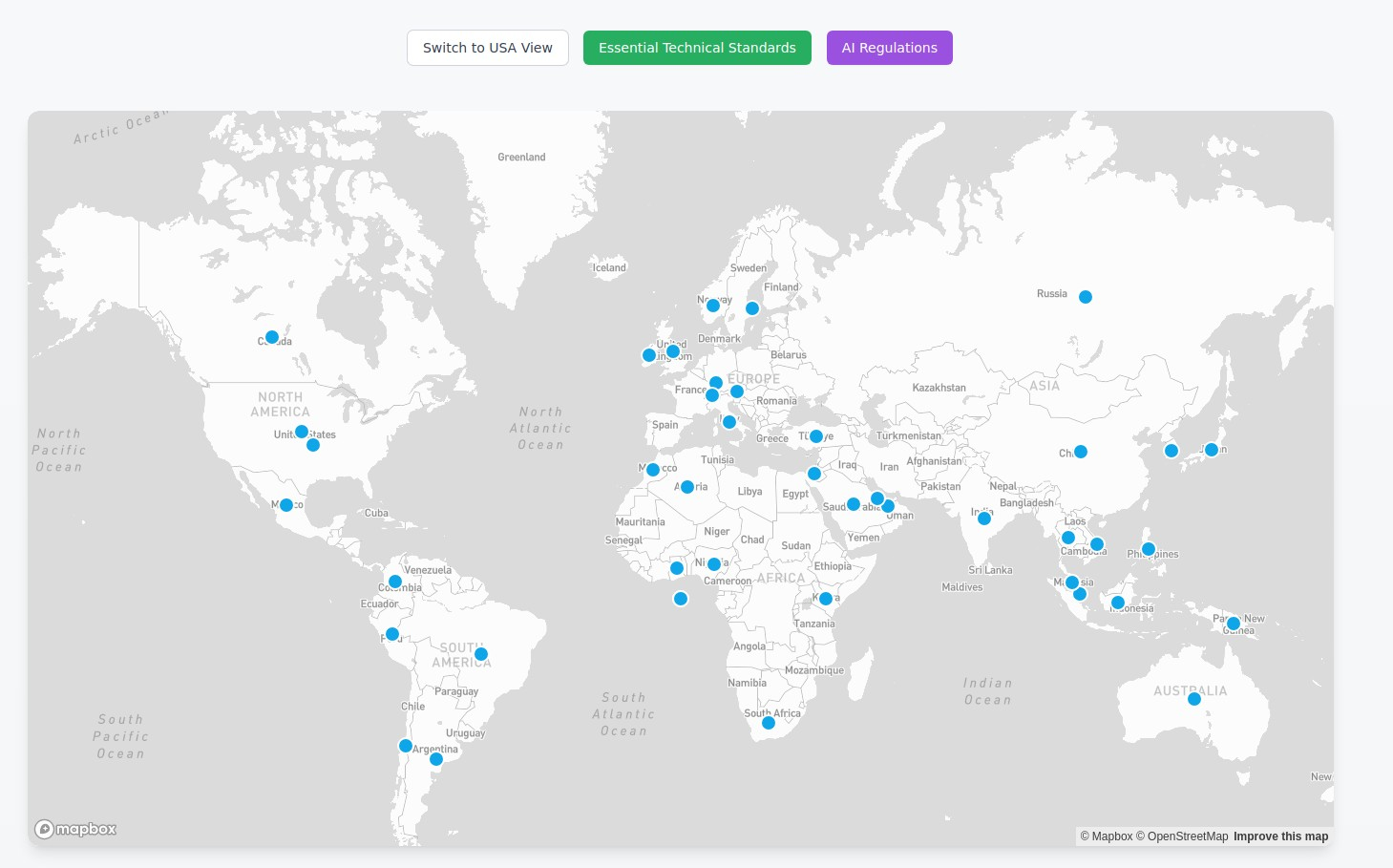
Protecting the data used to train and operate AI models is paramount for ensuring their integrity, reliability, and compliance with various regulations.
- Securing Training Data: AI models are only as good as their training data. Adversarial access can negatively impact this data, potentially hiding malicious activities or introducing vulnerabilities like model poisoning. Organizations must implement strict governance over data usage, secure sensitive data using appropriate techniques, and prevent unauthorized modifications.
- Avoiding Data Commingling: Leveraging enterprise data can enhance AI applications, but sensitive data should be sanitized or anonymized prior to use with Large Language Models (LLMs) to prevent potential data leakage or compliance violations. Differential privacy techniques, as referenced in the context of the Census Bureau, can be a relevant approach for anonymization.
- Limiting Sensitive Prompt Content: Attackers with unauthorized access to an organization’s prompts can infer sensitive information. Therefore, organizations should limit the inclusion of sensitive content in prompts and avoid using prompts to pass confidential information to LLMs, thereby reducing the risk of data exposure.
Access Controls: Governing Data Interactions
Effective access controls are fundamental to securing AI models and their associated infrastructure, especially in protecting the data.
- Principle of Least Privilege: Implementing the principle of least privilege is critical to ensure that only authorized individuals and processes have access to AI models, vector databases, and inference processes. This includes restricting both read and write operations on vector databases used in Retrieval-Augmented Generation (RAG) architectures.
- Strong Authentication and Authorization: Organizations must implement robust authentication, authorization, and monitoring mechanisms to prevent unauthorized access and model tampering, which could lead to data breaches or the introduction of malicious elements.
- Vector Database Security: Augmentation data in vector databases, crucial for RAG systems, can be a significant source of risk if not properly secured. Beyond access controls, data stored in these databases should be treated as sensitive and protected against tampering, which could lead to misleading or dangerous outputs from LLMs. Secure upload pipelines, logging and auditing of changes, and validation mechanisms should be implemented. Encryption at rest and in transit, along with digital signing of documents, can enhance trust in this layer.
Governance, Risk, and Compliance (GRC) in AI Data Management
Aligning AI initiatives with industry regulations and establishing governance frameworks are crucial for responsible and compliant AI usage.
- Establishing Governance Frameworks: Organizations must establish governance frameworks that align with industry standards and legal requirements, considering the evolving regulatory landscape surrounding AI. This may involve creating an AI GRC board or incorporating AI usage into an existing one.
- AI Risk Management: Implementing risk-based decision-making processes is essential for securely deploying AI systems. Understanding potential risks like unauthorized interactions, data tampering, data leakage, model poisoning, and prompt injection is critical for mitigation.
- AI Bill of Materials (AIBOM): Maintaining an AIBOM, modeled after software bill of materials (SBOM), can provide better visibility into the AI supply chain, including dataset and model provenance. While AIBOMs contain technical details useful to adversaries, their creation and maintenance are important for understanding and managing risks associated with data and models.
- Regulatory Adherence: Organizations must be aware of and adhere to evolving regulatory standards like the EU AI Act and other relevant frameworks listed in Table 1, such as the ELVIS Act and Executive Order 14110 in the US. Failure to comply with legal or regulatory mandates can prove costly. Tracking and adhering to non-mandated frameworks like the NIST AI Risk Management Framework and OWASP Top 10 for LLM can also be beneficial.
Monitoring and Auditability of AI Data Usage
Continuous monitoring and evaluation of AI systems are crucial for maintaining data integrity, detecting vulnerabilities, and ensuring compliance.
- Incorporating AI Monitoring: AI monitoring should be integrated into existing security policies to track data access patterns, identify anomalies, and detect potential security incidents.
- Protecting Audit Logs: Audit logs related to AI data access and usage may contain sensitive information and must be protected from unauthorized access and tampering.
- Tracking Refusals: Monitoring and tracking refusals from AI models can provide insights into potential security threats or policy violations.
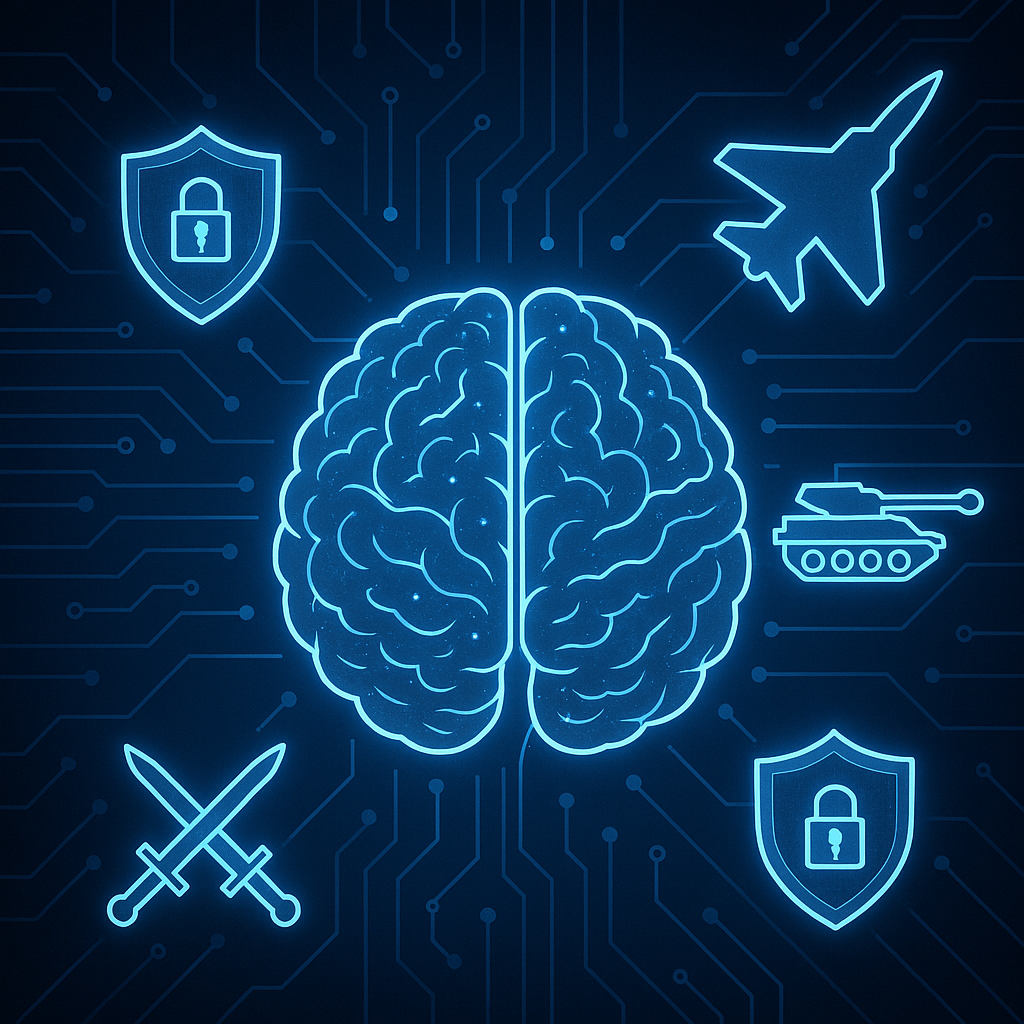
Secure Deployment Strategies and Data Residency
Decisions regarding AI model deployment (local vs. cloud) have security and compliance implications for data.
- Considering Legal Requirements: When choosing deployment options, organizations must carefully consider and codify legal requirements in contracts, especially concerning data usage, retention, and logging by third-party providers. Understanding where data will reside and how it will be controlled is crucial for compliance with data residency regulations.
- AI in Integrated Development Environments (IDEs): The integration of LLMs into IDEs can increase developer efficiency but may also inadvertently expose proprietary algorithms, models, API keys, and datasets. Organizations should explore IDEs with local-only LLM integrations when available to mitigate the risk of sensitive data exposure.
By proactively addressing these technical compliance considerations related to data, organizations can enhance the security and trustworthiness of their AI implementations, minimize risks, and ensure adherence to evolving regulatory requirements in this rapidly advancing field.