Advancing Trustworthy and Responsible AI: Insights from NIST’s AI 100-2E2023 Initiative
As artificial intelligence continues to transform industries—from healthcare and finance to transportation and cybersecurity—the need for robust, ethical, and reliable AI systems has never been more critical. The National Institute of Standards and Technology (NIST) is at the forefront of this transformation, pioneering efforts to establish a framework for trustworthy and responsible AI. The recent NIST AI 100-2E2023 initiative underscores this commitment, setting the stage for a future where AI systems are developed and deployed with transparency, accountability, and fairness.
The Imperative for Trustworthy AI
In today’s data-driven landscape, AI systems are often entrusted with decisions that have profound impacts on human lives. However, this growing reliance on AI also brings challenges:
- Bias and Fairness: Unchecked algorithms can inadvertently perpetuate or amplify existing social biases.
- Transparency: Complex AI models, particularly deep learning systems, often operate as “black boxes,” making it difficult for stakeholders to understand decision-making processes.
- Security and Robustness: As AI becomes more integral to critical infrastructure, ensuring its resilience against adversarial attacks and system failures is paramount.
- Accountability: Clear guidelines are necessary to determine who is responsible when AI systems fail or cause harm.
NIST’s work is driven by the recognition that ensuring the trustworthiness of AI is not just a technical challenge but a societal imperative.
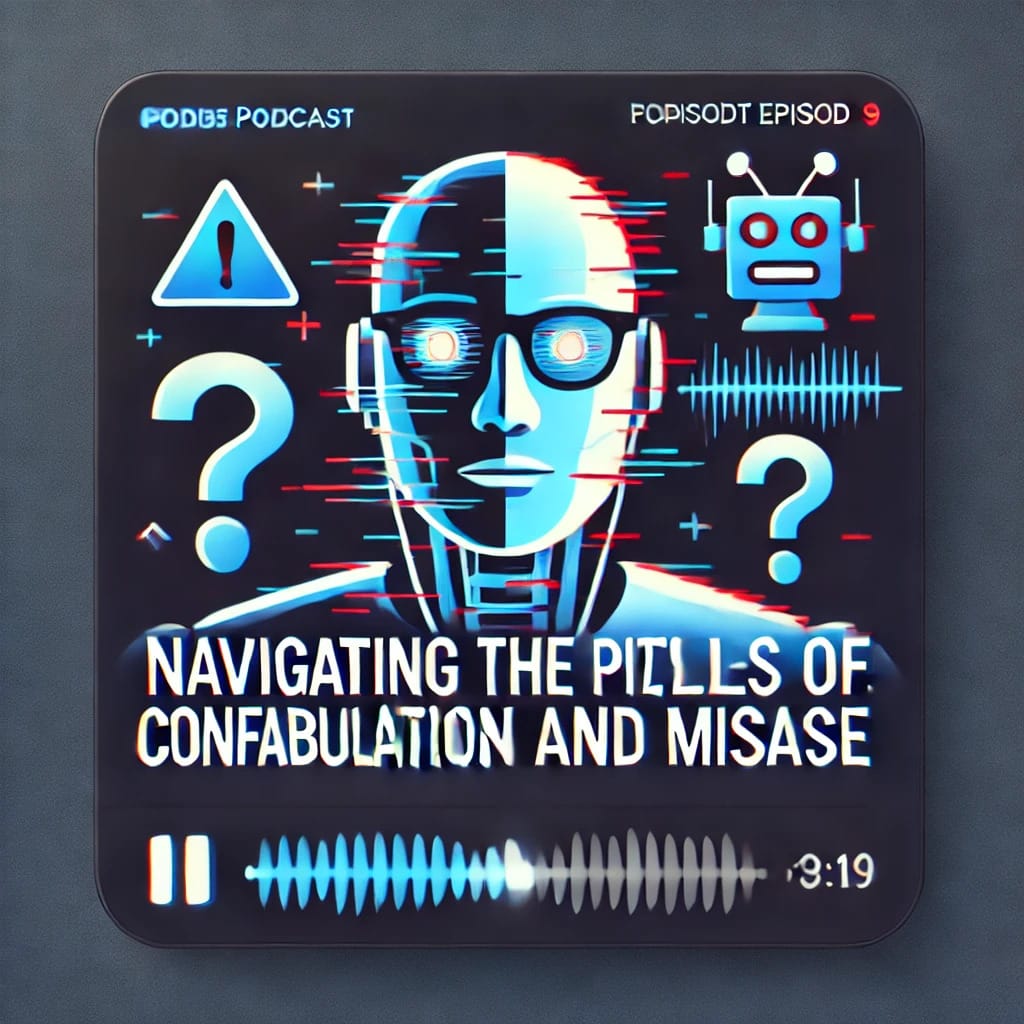
NIST’s Approach to Responsible AI
NIST’s strategy centers on developing a flexible, voluntary framework that guides organizations in assessing and mitigating risks associated with AI. Rather than prescribing a one-size-fits-all solution, the NIST AI Risk Management Framework (AI RMF) emphasizes:
- Outcome-Focused Practices: The framework is designed to be technology-agnostic, encouraging organizations to focus on the outcomes—such as safety, fairness, and reliability—rather than on specific technical implementations.
- Iterative Improvement: Recognizing that AI is an evolving field, NIST promotes continuous refinement and adaptation of standards as new challenges and technologies emerge.
- Collaborative Development: NIST works closely with industry leaders, academia, and other stakeholders to ensure that the framework reflects the diverse perspectives and needs of the AI ecosystem.
Key Pillars of Trustworthy AI
Central to NIST’s initiative are several core principles that define what it means for AI to be both trustworthy and responsible:
- Transparency and Explainability: Stakeholders should be able to understand how and why AI systems make decisions. This transparency fosters trust and enables better oversight.
- Fairness and Inclusivity: AI systems must be designed to avoid bias and ensure equitable outcomes. This involves rigorous testing and validation to prevent the reinforcement of societal inequalities.
- Robustness and Security: To maintain reliability, AI systems must be resilient against errors, adversarial attacks, and unexpected conditions. Robustness is achieved through comprehensive risk assessments and proactive mitigation strategies.
- Privacy and Data Governance: Responsible AI requires strict adherence to data protection standards, ensuring that personal and sensitive information is handled with care and integrity.
- Accountability: Clear lines of responsibility must be established, ensuring that both developers and deployers of AI systems can be held accountable for their performance and impact.
Spotlight on NIST AI 100-2E2023
The NIST AI 100-2E2023 initiative represents a significant milestone in the agency’s ongoing commitment to establishing trusted AI ecosystems. This initiative serves as a convergence point for the latest research, standards, and practices in AI risk management. Key highlights include:
- Enhanced Framework Guidance: The initiative builds on previous efforts by incorporating feedback from a broad spectrum of stakeholders, ensuring that the AI RMF remains relevant and practical.
- Focus on Real-World Applications: By emphasizing case studies and industry-specific challenges, NIST is bridging the gap between theoretical best practices and actionable guidelines.
- Global Collaboration: Recognizing that AI challenges are not confined by borders, the initiative encourages international cooperation to harmonize standards and foster a globally consistent approach to trustworthy AI.
Looking Ahead: The Future of AI Standardization
NIST’s work on trustworthy and responsible AI is dynamic and forward-thinking. As the technology landscape evolves, so too will the frameworks and standards that guide it. By championing transparency, fairness, and accountability, NIST is laying the groundwork for an AI future where innovation does not come at the expense of ethics or public trust.
The NIST AI 100-2E2023 initiative is not just a policy document—it’s a call to action for all stakeholders in the AI community. By embracing these standards, organizations can better navigate the complex ethical and technical challenges of modern AI, ensuring that this transformative technology benefits society as a whole.
In summary, NIST’s commitment to advancing trustworthy and responsible AI through initiatives like AI 100-2E2023 provides a clear roadmap for addressing the critical challenges facing AI today. With robust frameworks and collaborative efforts, the vision of a secure, fair, and transparent AI-powered future is within reach.
Additional Resources
Here are some additional resources that can enhance your article on advancing trustworthy and responsible AI, based on NIST's AI 100-2E2023 initiative:
NIST AI 100-2E2023 Report
The NIST AI 100-2E2023 report, titled "Adversarial Machine Learning: A Taxonomy and Terminology of Attacks and Mitigations," is a crucial resource for understanding the latest developments in AI security and trustworthiness[11]. This report develops a taxonomy of concepts and defines terminology in the field of adversarial machine learning (AML), which is essential for addressing potential vulnerabilities in AI systems[20].
NIST AI Risk Management Framework (AI RMF)
The NIST AI Risk Management Framework (AI RMF) is a cornerstone of NIST's approach to trustworthy AI[16]. It provides a structured approach for organizations to assess and mitigate risks associated with AI systems. The framework is designed to be voluntary and adaptable to various industries and use cases[5].
AI RMF Playbook
NIST has released an AI RMF Playbook, which offers practical guidance for implementing the AI Risk Management Framework[19]. This resource provides actionable steps and best practices for organizations looking to enhance the trustworthiness of their AI systems.
Trustworthy and Responsible AI Resource Center
NIST has launched the Trustworthy and Responsible AI Resource Center (AIRC), which serves as a comprehensive platform for accessing AI-related resources, including technical documents, toolkits, and case studies[18][50]. This center facilitates the implementation of and alignment with the AI RMF.
Case Studies and Use Cases
NIST provides documented use cases of organizations implementing the AI RMF, which can offer valuable insights into real-world applications of trustworthy AI principles[31]. These examples demonstrate how different sectors are addressing AI governance challenges.
AI Governance Best Practices
Several sources offer insights into AI governance best practices, which align with NIST's approach to trustworthy AI. These include establishing clear policies, ensuring transparency, and implementing robust monitoring systems[55][58].
Ethical Considerations
Resources discussing ethical challenges in AI, such as bias, privacy, and transparency, can provide context for the importance of NIST's work[41][42][45]. These discussions highlight the societal implications of AI development and deployment.
By incorporating these resources, you can provide a more comprehensive overview of NIST's efforts in advancing trustworthy and responsible AI, as well as the broader context of AI governance and ethics in which the AI 100-2E2023 initiative operates.
Citations:
[1] https://ama.drwhy.ai/nist-ai-risk-management-framework-ai-rmf.html
[2] https://www.schellman.com/blog/cybersecurity/nist-ai-risk-management-framework-explained
[3] https://airc.nist.gov/airmf-resources/airmf/
[4] https://centriconsulting.com/news/blog/nist-ai-risk-management-framework/
[5] https://blog.rsisecurity.com/comparing-nist-ai-rmf-with-other-ai-risk-management-frameworks/
[6] https://www.wiz.io/academy/nist-ai-risk-management-framework
[7] https://venafi.com/blog/ai-under-fire-new-nist-report-details-adversarial-machine-learning-aml-attack-types-and-mitigations/
[8] https://cltc.berkeley.edu/publication/a-taxonomy-of-trustworthiness-for-artificial-intelligence/
[9] https://nvlpubs.nist.gov/nistpubs/ai/nist.ai.100-1.pdf
[10] https://www.nist.gov/itl/ai-risk-management-framework
[11] https://nvlpubs.nist.gov/nistpubs/ai/NIST.AI.100-2e2023.pdf
[12] https://www.nist.gov/trustworthy-and-responsible-ai
[13] https://www.paloaltonetworks.com/cyberpedia/nist-ai-risk-management-framework
[14] https://nvlpubs.nist.gov/nistpubs/ai/NIST.AI.100-2e2023.ipd.pdf
[15] https://www.hunton.com/privacy-and-information-security-law/nist-releases-new-framework-for-managing-ai-and-promoting-trustworthy-and-responsible-use-and-development
[16] https://www.nist.gov/itl/ai-risk-management-framework
[17] https://csrc.nist.gov/pubs/ai/100/2/e2023/ipd
[18] https://content.govdelivery.com/accounts/USNIST/bulletins/351caae
[19] https://www.nist.gov/itl/ai-risk-management-framework/nist-ai-rmf-playbook
[20] https://csrc.nist.gov/pubs/ai/100/2/e2023/final
[21] https://www.responsible.ai/understanding-the-national-institute-of-standards-and-technology-nist-ai-risk-management-framework/
[22] https://www.brookings.edu/articles/nists-ai-risk-management-framework-plants-a-flag-in-the-ai-debate/
[23] https://csrc.nist.gov/News/2024/nist-releases-adversarial-ml-taxonomy-terminology
[24] https://blog.workday.com/en-us/the-new-nist-ai-framework-accelerating-trustworthy-ai.html
[25] https://www.auditboard.com/blog/a-checklist-for-the-nist-ai-risk-management-framework/
[26] https://www.restack.io/p/2024-eu-ai-regulations-knowledge-nist-answer
[27] https://connectontech.bakermckenzie.com/the-growing-importance-of-the-nist-ai-risk-management-framework/
[28] https://www.wolterskluwer.com/en/expert-insights/conducting-nist-audits-using-nist-ai-risk-management-framework
[29] https://www.nist.gov/document/workday-nist-ai-rmf-success-story
[30] https://airc.nist.gov/nist-ai-public-working-groups/
[31] https://airc.nist.gov/airmf-resources/usecases/
[32] https://www.holisticai.com/blog/nist-ai-risk-management-framework-playbook
[33] https://tentacle.co/blog/post/nist-ai-rmf-use-cases
[34] https://www.techtarget.com/searchsecurity/tip/How-to-use-the-NIST-CSF-and-AI-RMF-to-address-AI-risks
[35] https://www.nature.com/articles/s41599-024-02894-w
[36] https://www.coe.int/en/web/human-rights-and-biomedicine/common-ethical-challenges-in-ai
[37] https://bigid.com/blog/what-is-ai-governance/
[38] https://www.nlc.org/article/2023/10/10/the-ethics-and-governance-of-generative-ai/
[39] https://www.techtarget.com/searchenterpriseai/tip/Generative-AI-ethics-8-biggest-concerns
[40] https://www.zendata.dev/post/ai-governance-101-understanding-the-basics-and-best-practices
[41] https://news.harvard.edu/gazette/story/2020/10/ethical-concerns-mount-as-ai-takes-bigger-decision-making-role/
[42] https://www.mdpi.com/2227-9709/11/3/58
[43] https://www.fisherphillips.com/en/news-insights/ai-governance-101-10-steps-your-business-should-take.html
[44] https://www.gartner.com/en/articles/ai-ethics
[45] https://www.forbes.com/sites/eliamdur/2024/01/24/6-critical--and-urgent--ethics-issues-with-ai/
[46] https://www.isaca.org/resources/news-and-trends/isaca-now-blog/2024/the-key-steps-to-successfully-govern-artificial-intelligence
[47] https://drata.com/blog/new-nist-ai-rmf
[48] https://cset.georgetown.edu/article/translating-ai-risk-management-into-practice/
[49] https://www.skadden.com/insights/publications/2023/05/evaluating-and-managing-ai-risk-using-the-nist-framework
[50] https://airc.nist.gov
[51] https://annenberg.usc.edu/research/center-public-relations/usc-annenberg-relevance-report/ethical-dilemmas-ai
[52] https://www.snowflake.com/trending/ai-governance-best-practices/
[53] https://www.unesco.org/en/artificial-intelligence/recommendation-ethics
[54] https://dialzara.com/blog/10-common-ethical-issues-in-ai-and-solutions/
[55] https://www.ibm.com/think/topics/ai-governance
[56] https://www.who.int/news/item/18-01-2024-who-releases-ai-ethics-and-governance-guidance-for-large-multi-modal-models
[57] https://www.xenonstack.com/blog/ethical-ai-challenges-and-architecture
[58] https://www.domo.com/glossary/ai-governance